Active ingredients in drugs need to be carefully formulated with other chemicals called excipients – to optimize their properties and make them more effective medicines. This can help make the product more soluble, for example, allowing a drug to be formulated as an oral tablet rather than an injection, which patients may prefer – helping them to stick to their treatment.
Crystallizing a drug compound together with another chemical can improve its solubility – a process called co-crystallization. However, figuring out which chemicals (co-formers) will successfully crystallize with an active ingredient can take formulation scientists a long time and a lot of trial and error. This might lead to effective active ingredients being shelved.
Our innovative AI-based tool can predict potential compatible co-formers, including previously overlooked compounds. This significantly shortens the formulation process and accelerates the successful delivery of new medicines to patients.
Did you know?
-
~1 bn
USD to bring a new drug to market [2]
-
>30%
is the drug development cost dedicated to formulation [2]
-
~70%
of potential drugs in development have poor solubility [1]
The Formulation Challenge
During the drug discovery process, after a promising active pharmaceutical ingredient (API) has been identified in laboratory tests, the next stage is to formulate the active compound into a mixture with desirable drug-like properties.
The two most-common challenges in formulation science are improving the solubility of drugs – the ability to dissolve in a solvent – and enhancing its stability. Solubility and stability are essential properties for medicines. As they ensure that the active ingredient reaches the desired concentration in the bloodstream and sustain a therapeutic impact over time long enough to have a therapeutic effect.
Formulating an API into a stable and soluble medicine is an increasing challenge because sophisticated drug discovery tools (such as our AI-based tool AIDDISONTM) are now being used to identify potential pharmaceutical ingredients. These highly efficient tools can rapidly help medicinal chemists to identify and test a large number of promising drug candidates, but many of these are complex chemical compounds that are hard to formulate and show low solubility.
Co-crystallization – Solving Solubility
A relatively new approach to improve the solubility of a promising API is co-crystallization – where it is combined with a pharmaceutically acceptable co-crystal former (co-former) to form a co-crystal [3] [4].
The resulting co-crystals have unique properties that can address challenges related to drug solubility, stability, and bioavailability – often resulting in multiple-times higher solubility than the free active agent – without altering the chemical identity of the API or reducing its biological activity.
Various factors can affect whether co-crystallization will be successful in solving solubility and stability challenges. One of these is selecting the right co-former.
“It can be difficult to choose co-formers because you need to be able to crystallize both compounds in the same crystal lattice, which means they need to be matched structurally and have similar solubilities when crystallizing from solution,” says David Luedeker, Principal Scientist, Solid Formulation. “This means certain structural features might work together well and others will not. The only way to find out is to test each potential combination by screening different co-formers – which takes time, uses a lot of chemical materials, and is expensive. Unfortunately, it often ends without finding a successful formulation.”
It’s also common for formulation scientists to use excipients they are most familiar with, which limits the different co-crystal combinations they can generate. Many more formulation options could be explored if there were an easy way to test them without incurring the time and cost of additional hands-on experiments.
“Although there are some guidelines to help guide co-former choice, these are somewhat rudimentary, and a more sophisticated approach is required,” says Luedeker. “We had a vision of overcoming this issue by developing an artificial intelligence-based tool (AI) where you can load in the chemical structure of the chemical entity you are working with and it will suggest many different co-formers to focus on, increasing the likelihood of obtaining a ‘hit’ in your screening experiments.”
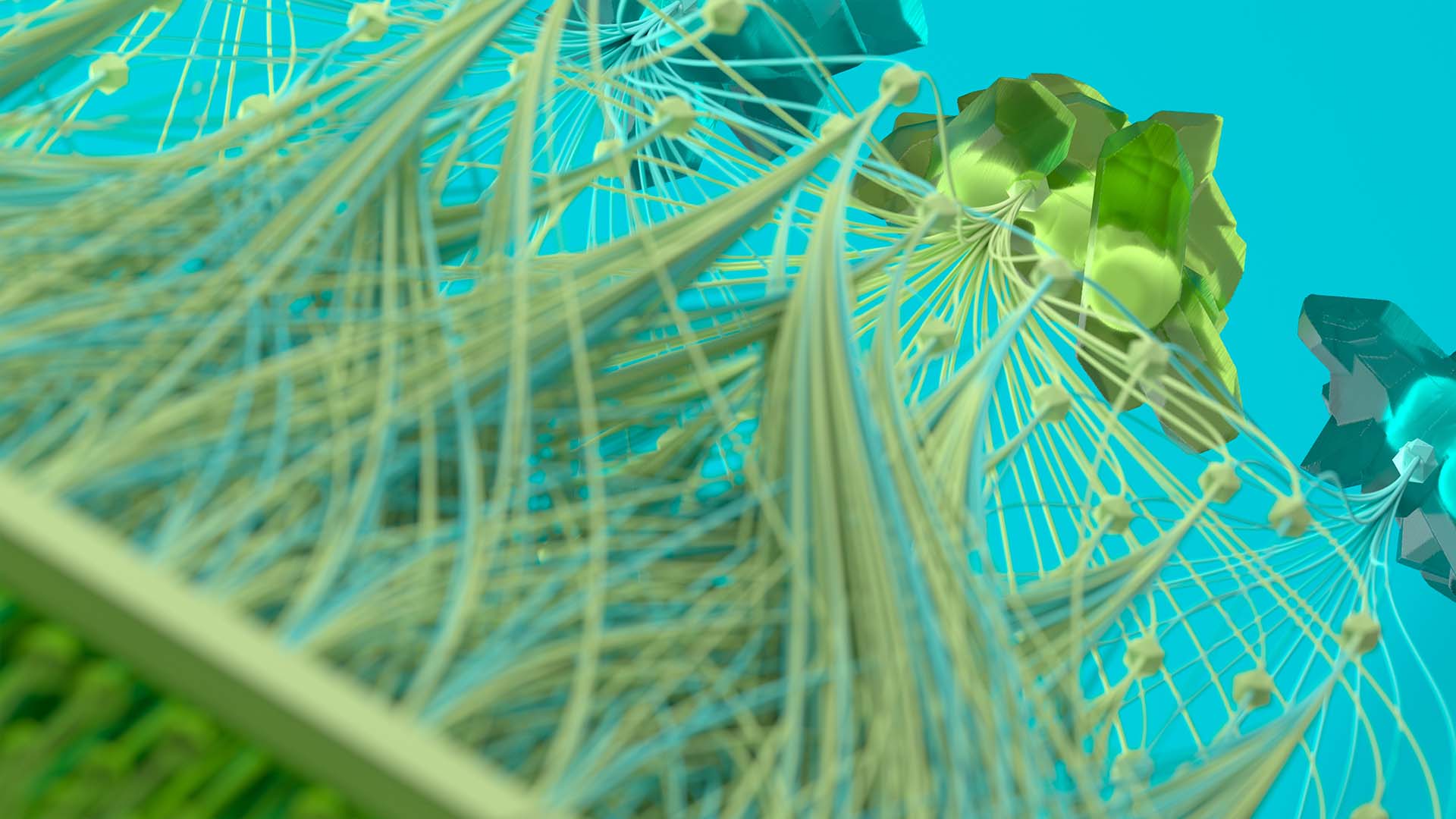
Developing a Predictive Formulation AI Tool
AI predictive tools are developed by using a set of training data that first teaches the computer model what it should be looking for (in this case, a likely successful co-former combination). Then, they use this “intelligence” to predict results with a brand-new, unseen data set.
Initial attempts to use publicly available co-crystal data to train the model were unsuccessful for two reasons: “First, the data in the literature were from various sources that used different measurements or methods for preparing co-crystals, including many inconclusive experiments,” explains Marko Hermsen, Lead Scientist, Digital Chemistry. “The other challenge is that we needed a tool for our customers who are working with compounds that no one has discovered yet, so we needed a very diverse training dataset of different types of APIs and co-formers for the model to be able to learn for any new type of drug or co-former it may encounter in the future.”
Diverse Training Data
There’s a well-known acronym in data science – GIGO: garbage in, garbage out – which means that if the quality of the data used to train an AI tool is poor, so will the results. For this reason, our scientists used a two-step process to train the model. The first step was to create a diverse set of compounds to train the AI tool.
“We started with a long list of more than 1,000 test drugs and co-formers that would be interesting candidates – systematically chosen to be very different from each other, and we categorized all these compounds within the model by their properties – such as melting point, shape, and weight – every property that would be related to their behavior to form co-crystals,” explains Hermsen. “We then used statistics to pick examples from this large set of co-formers and drugs that are very dissimilar to each other to create a highly diverse sub-set of training data.”
The resulting dataset covers a large chemical space, with potentially many different drug and co-former combinations that formulation scientists could encounter in the future. This means that the predictive tool can find co-formers that might not ever have been considered before by formulation scientists.
An Active Learning Approach
The second step was to gain as much information from the training dataset as possible by running experiments with different drug co-former combinations. This involved an “active learning approach”: a few drug-co-former combinations were chosen randomly to begin training the model. These generated predictions, but with some disagreement between the results. During the following stages, experiments were carried out using the drug/co-former combinations where the models had the highest disagreement.
“The idea is to not randomly sample possible drug and co-former combinations, which would take too long and cost too much, but to select those that promise the highest gain in information actively,” says Martin Fitzner, Senior Data Scientist, Digital Chemistry. “In practice, this meant that we collected new experimental data from the lab each week, used it to train the AI model, and then let the model decide which experiments should be run the following week by using the active learning approach.”
The team believes that the new AI tool performs better than other state-of-the-art models in the published literature, and it certainly performs better than the conventional approach of testing different compound and co-former combinations one-by-one experimentally.
“We think we can increase the chance of finding the right co-former by around three-fold,” says Luedeker. “So, with the same or fewer number of experiments, you will have a much higher and faster chance of finding a good co-crystal to take into development.”
How will This Tool Aid Drug Development?
It can take approximately USD 1 billion to bring a new drug to market, and about 30% of this cost is research related to formulation development.[2] Not only that, but fewer than 10% of drugs make it through development – many of them are shelved because they cannot be formulated into a medicinal product. [5]
With our predictive formulation tool, it becomes possible to formulate new APIs more efficiently and opens the door to resurrecting potentially effective drugs that were deprioritized because of formulation challenges.
“We can now offer a service to our customers where they provide us with their chemical compound data, and we run the predictive formulation tool and provide them with a package of results. This includes AI prediction alongside experimental data proving that AI-predicted co-formers produce good co-crystals,” says Luedeker. “What we’re seeing is that it’s not only customers in large pharmaceutical companies who are interested in accessing this tool, but smaller companies who cannot do extensive co-crystallization experiments and companies producing generic drugs (non-branded medicines) who want to improve the formulation of an existing product and overcome intellectual property challenges.”
“What’s rewarding about this project is that we had the chance to apply machine learning and data science techniques to everything from the ground up,” says Hermsen. “Usually, the way we work is to see what we can do with data that already exists, but here we created this from scratch and designed everything in the ideal way to deliver the best-possible machine learning model.”
Most importantly, this AI tool will ultimately benefit patients in several ways, from accelerating drug development to enabling more patient-friendly formulations of existing drugs, such as turning a drug that needs to be injected into the body into a pill that can instead be taken by mouth.
“As a solid-state chemistry scientist who’s worked on co-crystals for several years, I know how hard it is to screen and find new ones,” says Luedeker. “I’m delighted that we had the opportunity to develop something that is helping to solve this problem and could speed new medicines to patients faster.”
Our contribution
In 2012, the United Nations set out 17 Sustainable Development Goals (SDGs) that meet the urgent environmental, political, and economic challenges facing our world. Three years later, these were adopted by all member states. We are committed that our work will help to achieve these ambitious targets. Our in-house AI-powered predictive formulation tool fits under “Goal 9: Industries, Innovation and Infrastructure; Target 9.5: Enhance scientific research.” By harnessing the power of AI, our tool can help formulation scientists rapidly solve solubility and stability challenges for novel drugs, reducing the time and costs of drug development and getting new and better treatments to patients faster.
Learn more about SDGsReferences
[1] Ting JM, Porter WW 3rd, Mecca JM, Bates FS, Reineke TM. Advances in Polymer Design for Enhancing Oral Drug Solubility and Delivery. Bioconjugate Chem. 2018;29(4):939-952. doi:10.1021/acs.bioconjchem.7b00646
[2] Suresh, P., Basu, P.K. Improving Pharmaceutical Product Development and Manufacturing: Impact on Cost of Drug Development and Cost of Goods Sold of Pharmaceuticals. J Pharm Innov 3, 175–187 (2008). https://doi.org/10.1007/s12247-008-9043-1
[3] Bhatia, M and Devi S. Co-crystallization: A Green Approach for the Solubility Enhancement of Poorly Soluble Drugs; CrystEngComm, 2024,26, 293–311. Doi: 10.1039/D3CE01047C
[4] Essen CV, Luedeker D. In Silico Co-crystal Design: Assessment of the Latest Advances. Drug Discov Today. 2023;28(11):103763. doi:10.1016/j.drudis.2023.103763
[5] Sun D, Gao W, Hu H, Zhou S.; Why 90% of Clinical Drug Development Fails and How to Improve It? Acta Pharm Sin B. 2022;12(7):3049-3062. doi:10.1016/j.apsb.2022.02.002
Work With Us
Transforming Lives with Artificial Intelligence
Do you believe in the power of AI in drug development? By joining our team, you can help develop next-generation software that can accelerate the development of effective new drugs that can help transform patients’ lives.
View All Jobs Join our Talent Zone
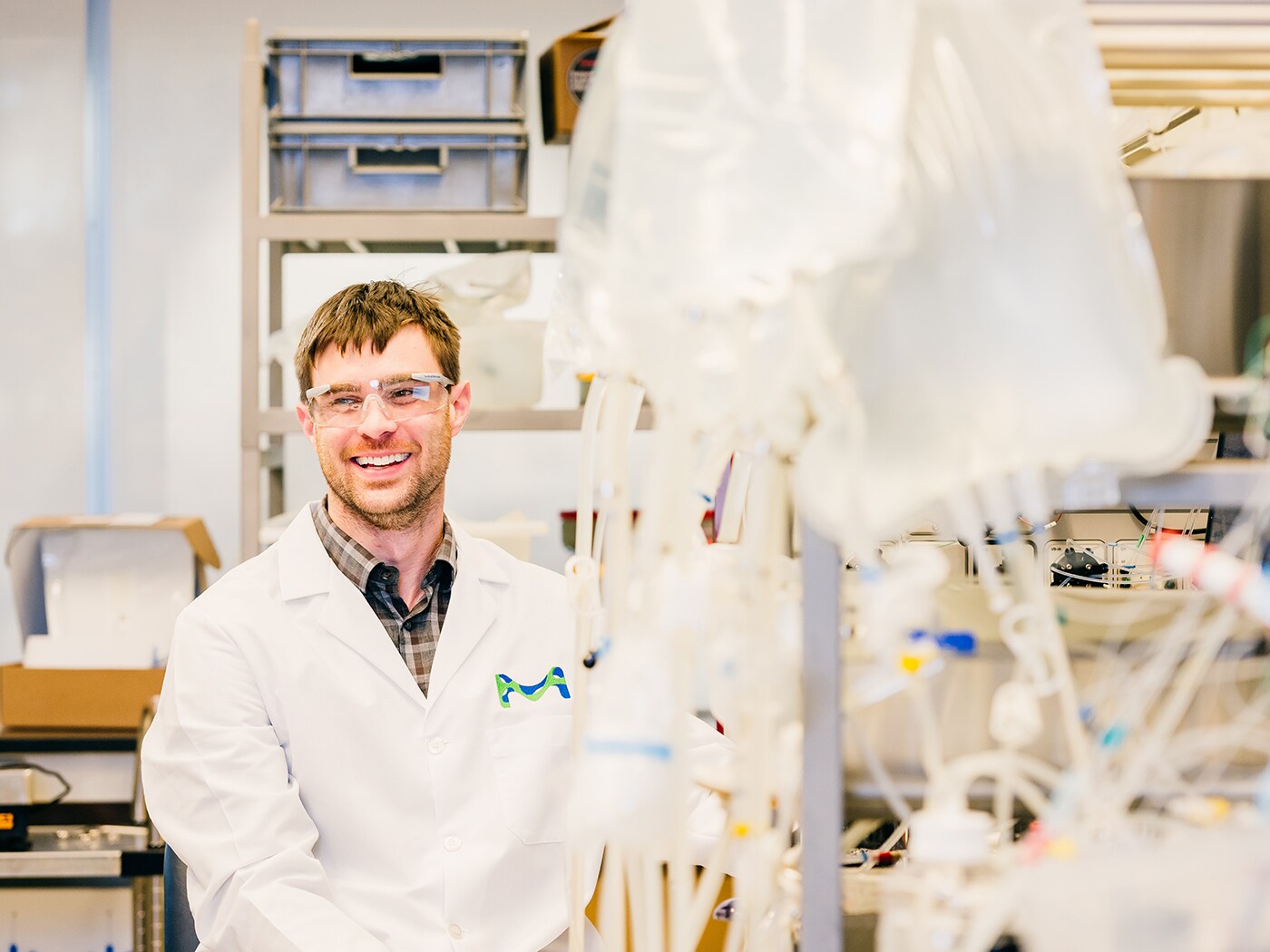