Machine learning in medicine
We still have no reliable way to monitor and measure many health conditions and chronic diseases. But new digital health support tools promise to revolutionize care.
Digital tools to improve wellbeing
A patient with a chronic illness, such as Parkinson’s disease, may only see a clinician who specializes in their condition (in this case, a neurologist) twice a year. Yet between those appointments, they are likely to experience ups and downs in their symptoms that could be managed better to improve their quality of life. Ideally, patients would benefit from being digitally connected in real-time to their specialists.
Bjoern Eskofier, Heisenberg-Professor for Computer Science at the Friedrich-Alexander-Universität Erlangen-Nuernberg (FAU), is developing digital tools to achieve just that. In 2020, together with Manager Magazin, we awarded our Curious Mind Award to Professor Eskofier for his work on digital support systems in sports and medicine. Among other projects, he uses machine learning and mobile sensors to analyze the gait of patients with Parkinson’s disease, improve diagnostics, and measure treatment success. A medical doctor can use the information provided by these digital tools to make more timely and personalized adaptations to the treatment of the patients.
Did you know?
-
six
months time between Parkinson’s disease check-ups
-
146
- or more precise 146.574 real-world strides used in evaluation [4]
-
94%
of four 10m walk tests successfully analyzed from at-home data [5]
Machine learning in medicine
Many of us are now familiar with ‘wearable’ technology such as activity trackers and smart watches. The continuous, ‘real-time’ data that these devices provide present a tremendous opportunity to better understand our health and wellbeing. But to achieve this, we need computer software tools that can look for patterns in these data that tell us something new or meaningful. This is the purpose of machine learning, where a computer is given a large dataset and is tasked with finding patterns without explicit instructions [1]. From this, it becomes possible to establish a set of rules (an algorithm) that the computer can use to identify similar trends in the future using new data. In this way, the computer can analyze data and make decisions – i.e., it has artificial intelligence (AI).
There are huge global efforts to use machine learning and AI to spot patterns in health and medical data to understand more about health and disease [2]. Many of these efforts are focused on using data from large numbers of people to spot overall trends for a specific disease, but in Professor Eskofier’s Machine Learning and Data Analytics lab, they are seeking to use these data in a highly personalized way.
“My vision is to build a better healthcare system through the use of digital tools,” says Eskofier. “If you can just make the life of a single chronically ill patient better, that's an achievement.”
His lab works on new ways to make unmeasurable diseases measurable. “Around 120 years ago, two inventions opened new diagnostic windows: the X-ray was invented by W.C. Röntgen and the electrocardiogram by W. Einthoven. Both discoveries, which made certain diseases visible and measurable for the first time, were later awarded with the Nobel prize in physiology or medicine. We are researching to make measurement possible for diseases like Morbus Parkinson by combining sensor technology with AI.”
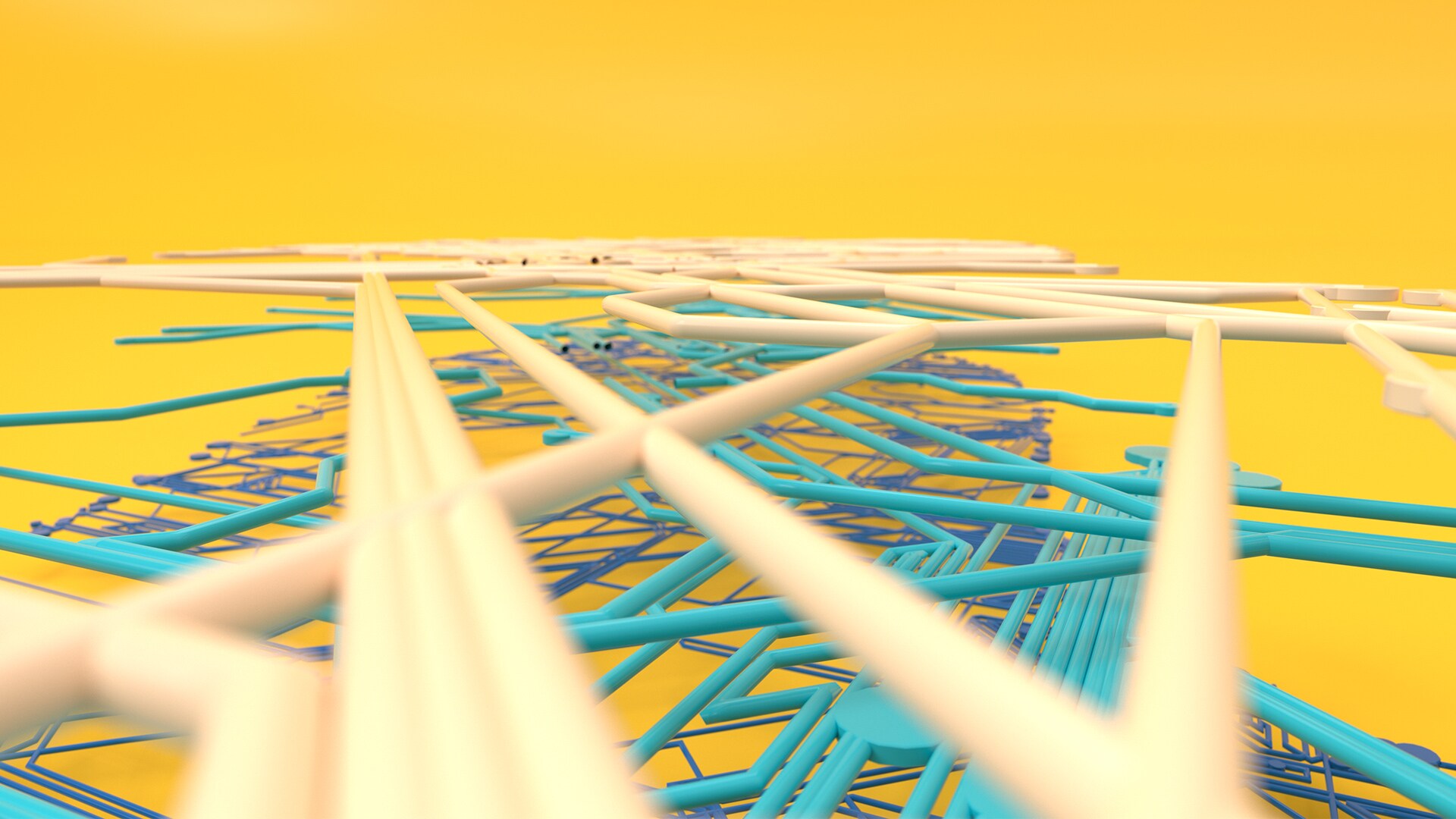
Progress against Parkinson’s disease
Disease progression and treatment response in Parkinson’s disease is usually evaluated with a standard questionnaire called the Unified Parkinson Disease Rating Scale, which assesses symptoms such as slowness of movement, muscle stiffness, involuntary tremors, and unsteady gait. But a key limitation is that these measurements are subjective.
“If a single patient goes through three different medical experts, you will get three different ratings,” says Eskofier, “But with a sensor, you can make the disease objectively measurable.”
Using a sensor that slips into the patient’s shoe, Eskofier’s lab, working in close collaboration with the University Hospital Erlangen, has built up a database of gait analysis from people with Parkinson’s disease and used this to create an algorithm that predicts symptom severity, called eGaIT in 2013 [3]. Nearly 700 gait features were used to build eGaIT, and when it was tested to see if it could predict disease severity in a new group of patients, it successfully distinguished PD patients from controls with 91% accuracy for the most severe patients [3]. The algorithm was subsequently improved, for example to work for real-world walking of patients [4,5].
“AI is always dependent on data quality, and we now have one of the largest available databases in Parkinson's gait analysis,” he explains. “Through a start-up company that I co-founded, we are now encoding this data knowledge and algorithm into a digital healthcare solution that will hopefully soon be available to every Parkinson's disease patient in Germany.”
The progress has been expedited by the new Digital Health Care Act in Germany, which means a sensor or app that is medically approved can now be prescribed by doctors and reimbursed through the health insurance system. “This was a big game-changer for startups like ours because before then it was unclear how you could get digital health tools reimbursed.”
It's exciting to think that this approach is not just limited to Parkinson’s disease, but to any conditions where real-time patient support could be helpful. “It could be an at-home ultrasound in pregnancy or an at-home heart function measurement for patients living with cardiac hypertrophy, “says Eskofier. “It always starts with a measurement, so we are looking at any area where sensors or wearables can tell us how a patient is doing at home, and how we can use that measurement to optimize their care.”
More than just algorithms
It’s essential that any digital health technology provides meaningful insights and is accessible to the end-user, which means everyone in Eskofier’s lab needs some medical understanding. “All my Ph.D. students must work in the hospital if they work on a clinical medical question because we need to understand how the patient lives with a condition and how the doctor treats it. It's about embedding the algorithm in the healthcare domain you're working in.”
Usability by healthcare professionals and patients is also essential. “Lots of people can write algorithms, but it’s essential to take the perspective of the user,” says Eskofier. “This can be hard when you are knowledgeable about how the sensor and the algorithm works, but it doesn't help anyone if the patient and/or healthcare expert cannot understand how to use and interpret the tool.”
A further ingredient for successful digital health is trust, and many people are understandably skeptical about sharing any type of personal data. “If I ask you how many different entities your personal address have, you probably couldn’t tell me. Already much of our data is being shipped into a digital void without us feeling able to track what is happening with it. But you're entitled by European GDPR law to know who is using your digital data,” says Eskofier. “So, we are developing ‘personal health data spaces’ where people can see exactly who is accessing their data, what publications and studies were done with it, and providing the option to retract it at any time.”
Next-generation sensors
Across their many projects, Eskofier’s team either adapts commercially available sensors and wearables or develop their own to meet a specific need, such as the shoe sensor for gait analysis. But they are also co-developing new sensor concepts that could be transformational for monitoring health and wellbeing. Based on fundamental physics, the sensor measures minuscule changes in the electromagnetic fields created by wireless routers within the home when a human – mostly made up of water – walks through it.
“This means that gross movements like walking and gestures can be measured, as well as micro-movements like the twitching of muscles under the skin that reveal our expressions and nano-movements like sweat building on the skin,” says Eskofier. “However, even if you can measure sweat glands emitting water or sweat, we don’t yet know what this means. So, we are testing the approach in various use cases. For example, in people with and without depression, we want to understand how movement and expressions change depending on the mood, so we could potentially use these measurements as a signal to health care experts when someone needs more support.”
This touch-free, non-invasive sensor model is also being explored in stress, Parkinson's, palliative care, and rheumatology - all conditions targeted in their research center, EmpkinS. But the technology could be used for many other applications, says Eskofier.
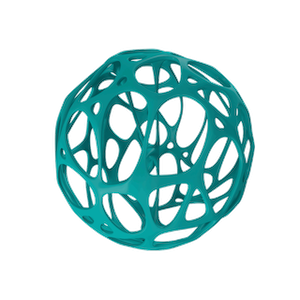
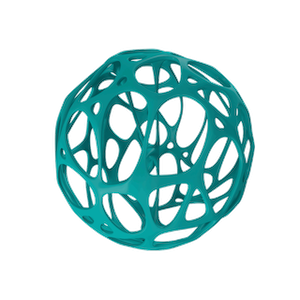
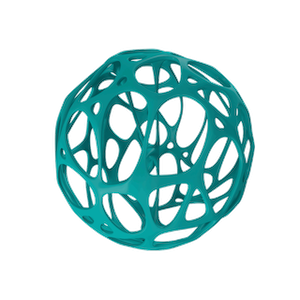
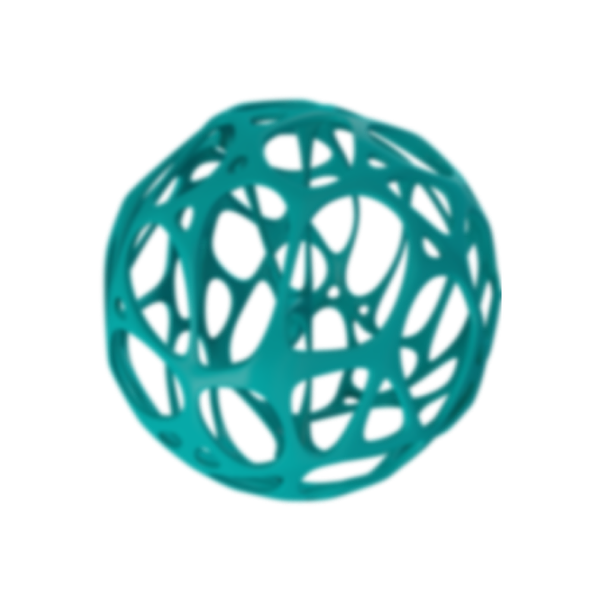
“The Curious Mind Researcher Award honors those scientists whose work is characterized by originality and excellence. Their innovative spirit is providing impetus for the future viability of Germany and Europe as an innovation hotspot.”
The Curious Mind Award
For progress in advancing these innovative digital health tools, Eskofier was awarded our Curious Mind award in the "Life Science" category. The Curious Mind Award recognizes young Germany-based scientists under the age of 40 whose work not only demonstrates the highest level of academic excellence, but also indicates that it will provide an impetus for the future of the global economy.
On receiving his award, Eskofier said: “I'm grateful to Merck KGaA, Darmstadt, Germany and Manager Magazin for putting out this award because it has had a tremendous impact. It's a door opener and has created lots of visibility for my work. I can only emphasize how important this is for a career.”
Our contribution
In 2012, the United Nations set out 17 Sustainable Development Goals (SDGs) that meet the urgent environmental, political and economic challenges facing our world. Three years later, these were adopted by all member states. We are committed that our work will help to achieve these ambitious targets. Bjoern Eskofier’s award-winning work on digital health tools fits under ‘Goal 3 – Ensure healthy lives and promote well-being for all at all ages; Target 3.4: By 2030, reduce by one third premature mortality from non-communicable diseases through prevention and treatment and promote mental health and well-being.
Learn more about SDGsReferences
[1] Bzdok, D., Krzywinski, M. & Altman, N. Machine learning: a primer. Nat Methods 14, 1119–1120 (2017). https://doi.org/10.1038/nmeth.4526
[2] Rajpurkar, P., Chen, E., Banerjee, O. et al. AI in health and medicine. Nat Med 28, 31–38 (2022). https://doi.org/10.1038/s41591-021-01614-0
[3] Klucken J, Barth J, Kugler P, et al. Unbiased and mobile gait analysis detects motor impairment in Parkinson's disease. PLoS One. 2013;8(2):e56956. doi:10.1371/journal.pone.0056956
[4] Roth N, Küderle A, Ullrich M, Gladow T, Marxreiter F, Klucken J, Eskofier BM, Kluge F. Hidden Markov Model based stride segmentation on unsupervised free-living gait data in Parkinson's disease patients. J Neuroeng Rehabil. 2021 Jun 3;18(1):93. doi: 10.1186/s12984-021-00883-7
[5] Ullrich M, Kuderle A, Hannink J, Din SD, Gasner H, Marxreiter F, Klucken J, Eskofier BM, Kluge F. Detection of Gait From Continuous Inertial Sensor Data Using Harmonic Frequencies. IEEE J Biomed Health Inform. 2020 Jul;24(7):1869-1878. doi: 10.1109/JBHI.2020.2975361.
Work With Us
Work with us on innovative digital technologies
Inspired by the potential of using data and digital tools to improve wellbeing? By joining our company, you will be part of a team at the forefront of developing new digital technologies to improve patient experience and treatment outcomes.
View All Jobs Join our Talent Zone
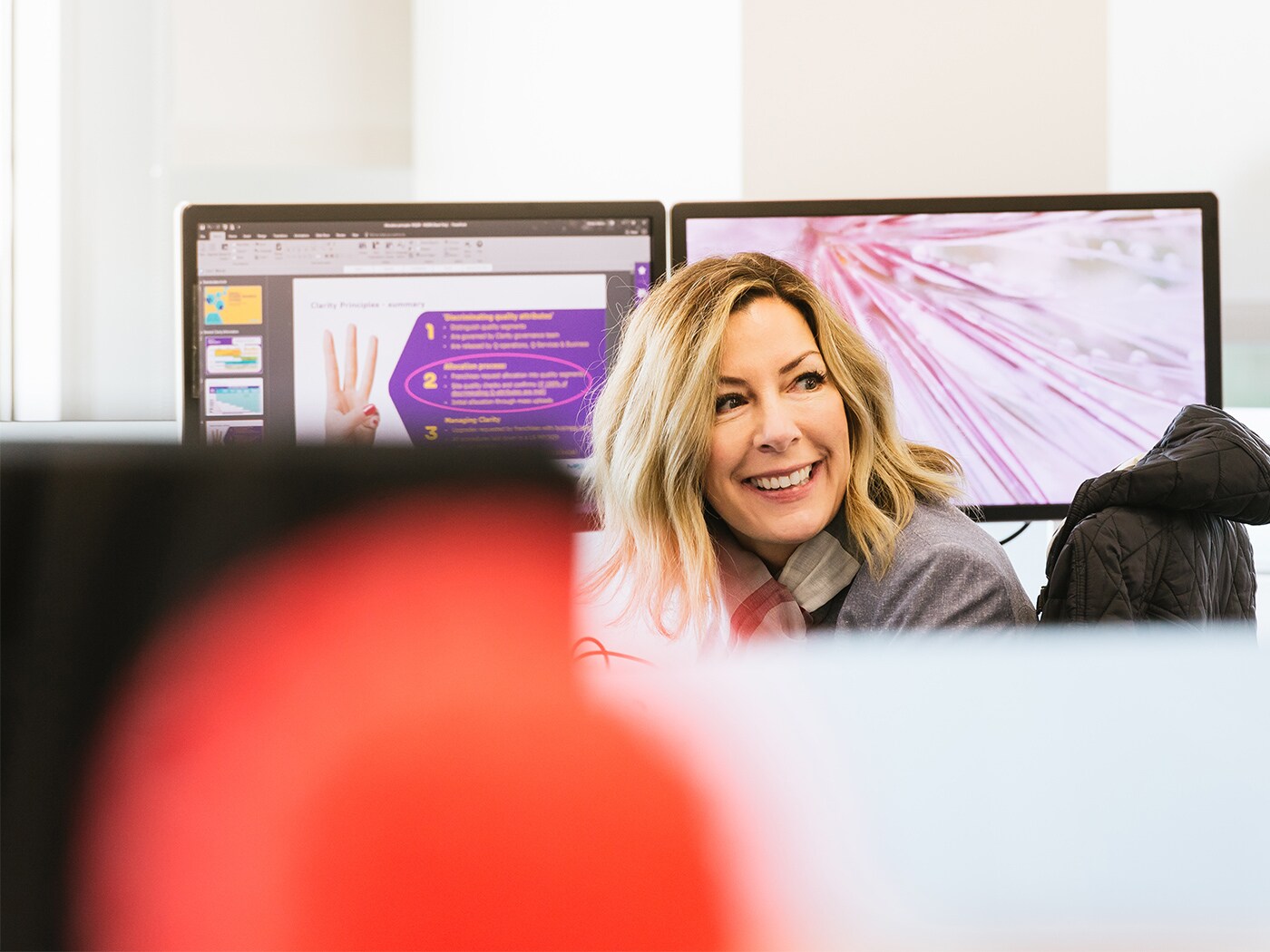